MICCAI Grand Challenge:
Prostate MR Image Segmentation 2012
October 1, 2012
Nice, France
Welcome to the website of the 'Prostate MR Image Segmentation'-challenge 2012. The goal of this challenge is to compare interactive and (semi)-automatic segmentation algorithms for MRI of the prostate. During MICCAI2012 the results of the challenge will be presented in conjuction with a live challenge, evaluating the algorithms on unseen data.
Update March 15th 2019
We have decided to change the requirements for submissions to the PROMISE12 Challenge. Over the past months we have noticed that groups are uploading multiple submissions with different deep learning architectures. Although most of these architectures are substantially different from each others (so adhering to the rules), this way of submitting results leads to 'training on the test set', which makes the leaderboard less meaningful. Optimization, so also architecture selection, should be performed on the training set only! To prevent excessive submissions, we are now requiring that each submission is accompanied by a publication on arXiv or any other official preprint server. You can add the URL during your submission. Submissions without a valid preprint URL or without a full description will no longer be evaluated. If you need your submission to be evaluated because you are submitting to a conference or journal which does not allow preprints, please send the anonymized paper to the challenge organizers via e-mail.
Update May 27th 2018
We have switched the PROMISE12 evaluation to a new system. We are working on reuploading all old methods to this new framework. Note that scores might have changed slightly due to the new way the boundary based metrics are calculated. This does not result in signficant changes in the ranking.
Update January 18th 2018
We have a new number 1 and number 2, both reaching scores above 87 points. See the results page for more details.
Update May 10th 2016
The paper from the Emory-group (currently ranking second) has been published and is available. See the results page. Furthermore, the PROMISE12-challenge has migrated to grand-challenge.org (https://github.com/comic/grand-challenge.org), if you have an old user account you will need to register again.
Update August 30th 2015
A new semi-automated method has been submitted by the CAMP-TUM-group, achieving 6nd place. See the results page.
Update July 21st 2015
A new semi-automated method has been submitted by the Emory-group, achieving 2nd place. See the results page.
Update March 30th 2015
A new method has been submitted by the Visifield-group and was added to the results page.
Update March 6st 2015
A new method has been submitted by the CIM@LAB-group and was added to the results page.
Update December 4th 2014
A new submission using a semi-automatic system by the group from the ETHZ, Zuric has been added to the results page.
Update November 26st 2014
A new submission by the CIM@LAB-group has been added to the results page.
Update January 21st 2014
The overview paper of the challenge has been published in Medical Image Analysis (paper). The challenge is still open for new submissions. If you want to publish a paper using the PROMISE12-data, it is mandatory to also submit your results on the test set through the challenge website. We urge reviewers to not accept papers without test set results.
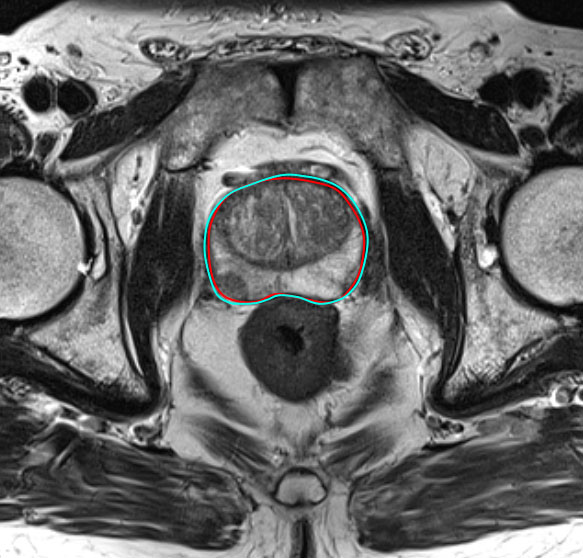
How does this challenge work?
Teams (research groups, companies, etc.) who are developing a segmentation algorithm can register and download multi-center, multi-vendor training data from this website to train their algorithms. Test data will also be supplied. The segmentation results of the algorithms on the test data can be submitted through this website, including a short paper explaining the algorithm. The segmentations will be automatically evaluated against the reference standard. The results of the algorithm will then be ranked and shown in the results section of this website. For more information please see the details section. The resultant evaluation measures will be published on this website and presented during MICCAI2012. At MICCAI2012 there will also be a live challenge, where participants will evaluate their algorithms live on new, unseen data. After MICCAI this website will remain online to allow the challenge to continue.
Why do we want to segment the prostate on MR images?
Determination of prostate volume (PV) facilitates an assessment of prostate disorders and, for prostate cancer, in conjunction with other parameters, can help predict the pathologic stage of disease, offers insights into the prognosis, and helps predict treatment response. Prostate-specific antigen (PSA) levels have been modified to derive the PSA density by incorporating PV calculations to help guide clinical decisions. The clinical value of prostate-specific antigen density, however, is dependent on the quality of the PV estimate. The accuracy and variability of PV determinations pose limitations to its usefulness in clinical practice. Information on the size/PV, shape, and location of the prostate relative to adjacent organs is also an essential part of surgical planning for prostatectomy, radiation therapy, and emerging minimally invasive therapies, such as cryotherapy and high-intensity focused ultrasound (HIFU). Recently, the high spatial resolution and soft-tissue contrast offered by MRI makes it the most accurate method available for obtaining this kind of information. This, combined with the potential of MRI to localize and grade prostate cancer, has led to a rapid increase in its adoption and increasing research interest in its use for this application. Furthermore, and of particular relevance to the MICCAI community, is the fact that accurate prostate MRI segmentation is an essential pre-processing task for computer-aided detection and diagnostic algorithms, as well as a number of multi-modality image registration algorithms, which aim to enable MRI-derived information on anatomy and tumor location and extent to aid therapy planning and guidance.
Consequently, there is a real clinical and research need for developing algorithms for (semi)-automated, computerized segmentation of the prostate in MRIs that offer robust, high quality results for a variety of clinical applications which are starting to incorporate MRI as part of routine clinical practice.